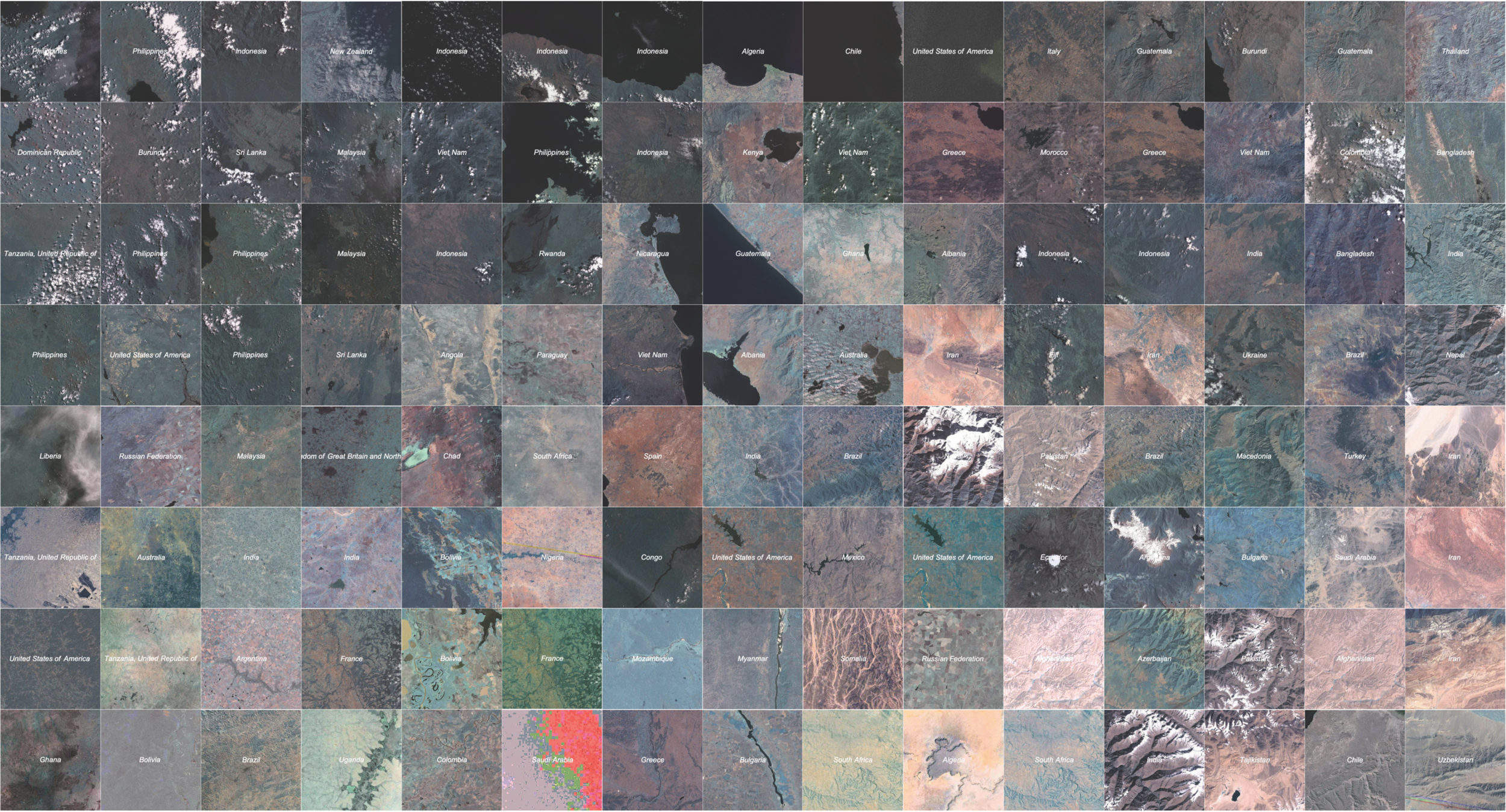
AN ML APPROACH FOR RAPID DISASTER RESPONSE BASED ON MULTI-MODAL DATA
The case of housing & shelter needs
In collaboration with Tina Comes
Along with climate change, more frequent extreme events, such as flooding and tropical cyclones, threaten the livelihoods and wellbeing of poor and vulnerable populations. One of the most immediate needs of people affected by a disaster is finding shelter. While the proliferation of data on disasters is already helping to save lives, identifying damages in buildings, assessing shelter needs, and finding appropriate places to establish emergency shelters or settlements require a wide range of data to be combined rapidly. To address this gap and make headway in comprehensive assessments, this paper proposes a machine learning workflow that aims to fuse and rapidly analyze multimodal data. This workflow is built around open and online data to ensure scalability and broad accessibility. Based on a database of 19 characteristics for more than 200 disasters worldwide, a fusion approach at the decision level was used. This technique allows the collected multimodal data to share a common semantic space that facilitates the prediction of individual variables. Each fused numerical vector was fed into an unsupervised clustering algorithm called Self-Organizing-Maps (SOM). The trained SOM serves as a predictor for future cases, allowing predicting consequences such as total deaths, total people affected, and total damage, and provides specific recommendations for assessments in the shelter and housing sector. To achieve such prediction, a satellite image from before the disaster and the geographic and demographic conditions are shown to the trained model, which achieved a prediction accuracy of 62%.
One of the critical challenges in disasters is combining the plethora of available data, from social media to satellite imagery and turning it into concrete and actionable insight. This is especially true for humanitarian housing and shelter needs. However, such operation deals with spatial and constructive constraints that, if not consider, can fail to achieve adequate solutions. Therefore, the objective of this experiment is to create a proof of concept workflow that can guide the responses of decision-makers at the operational level to address operations in the shelter and housing sector.
For this experiment we used 3 classes of data:
1. disaster characteristics
2. location-based risk
3. spatial conditions.
To ensure scalability and low cost of this approach, we only used open and online data. For the disaster characteristics, we used 202 disasters from the EM-DAT database from the year 2010-2019. For location-based risk, we include the World Bank’s Global Urban Risk Index, the European Commission INFORM index, UNdata and for climatic conditions, and Sea Temperature Conditions (STC) at the time of the disaster.
To capture the spatial conditions, we used satellite imagery from Google Maps. We analyzed spatial conditions and urban features from recognizing transportation networks; major infrastructures and characteristic features such as rivers and coasts as well as land use.
Since all the data collected by each disaster are from various sources, their modality is equally diverse. Therefore, to deal with this multimodality, we applied a fusion approach at the decision level. This technique allows the collected multimodal data to share a common semantic space that facilitates the prediction of individual variables.
To explore the relationships of the 202 disasters, we use a machine learning unsupervised clustering algorithm, called self organizing maps, which visualized the events as a similarity landscape, where each natural disaster is positioned according to the similarity of its weight value concerning its neighbors.
This trained self organizing maps can further visualize each feature describing a natural disaster to provide a detailed exploration. In this way, it is possible to identify which features influence the prediction the most. And we could selected features to be considered in further research.
To validate the workflow, data was used from 2020 on natural disasters. After feeding the new data to the trained self organizing maps, each data point found a node, which had the closest Euclidean distance to their numerical fused vector. By comparing the prediction with the ground truth labels. We found that of the 42 ground truth data points, 26 were correctly labeled, 7 were over predicted, and 9 were under predicted with only one degree more or one degree less, and the highest accuracy in the category number of affected people was 78% correct. However, in the face of a natural disaster, following the precautionary principle, overprediction – especially if it is with respect to only one of the categories – is more acceptable than underprediction. If we add the correct predictions with the over predictions, we arrive at a model that has 83% non-under prediction.
Additionally, to add specific information to facilitate decisions in the shelter and housing sector. We will focus on the information provided by The Global Shelter Cluster, which has published recommendations for the construction of shelters and temporary houses.
These recommendations can be translated into a list of potential assessments that will strengthen the response and identify assessments that may reduce the effectiveness of the response. The final prediction includes the impact that this disaster will have on the community and also show a list of recommendations on what to do and what not to do when making decisions in the shelter and temporary housing sector after a natural disaster.
This experiment allowed us to analyze a natural disaster as a part of a set of disasters that may show similarities according to the different characteristics considered. In the present case, general attributes of the population and the sector were considered… While the attributes selected do not fully capture the complexity of decisions in natural disasters, this approach provides a starting point to expand beyond the shelter & housing sector. The next step for us will be to apply this research in an actual case after a natural disaster to validate the effectiveness. So what we want is to invite humanitarian organizations to work in collaboration with us for future disaster response.
Gallery
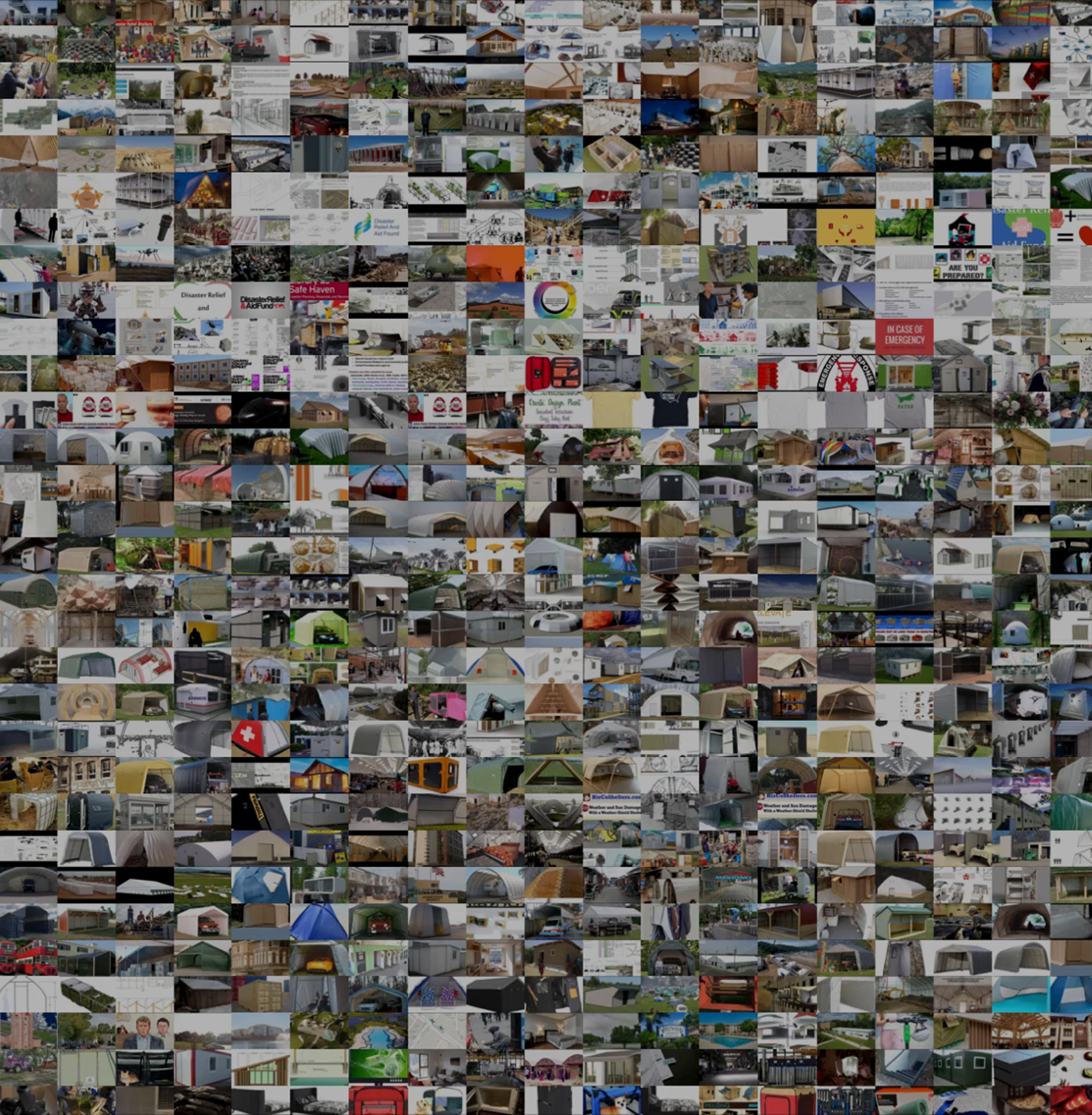
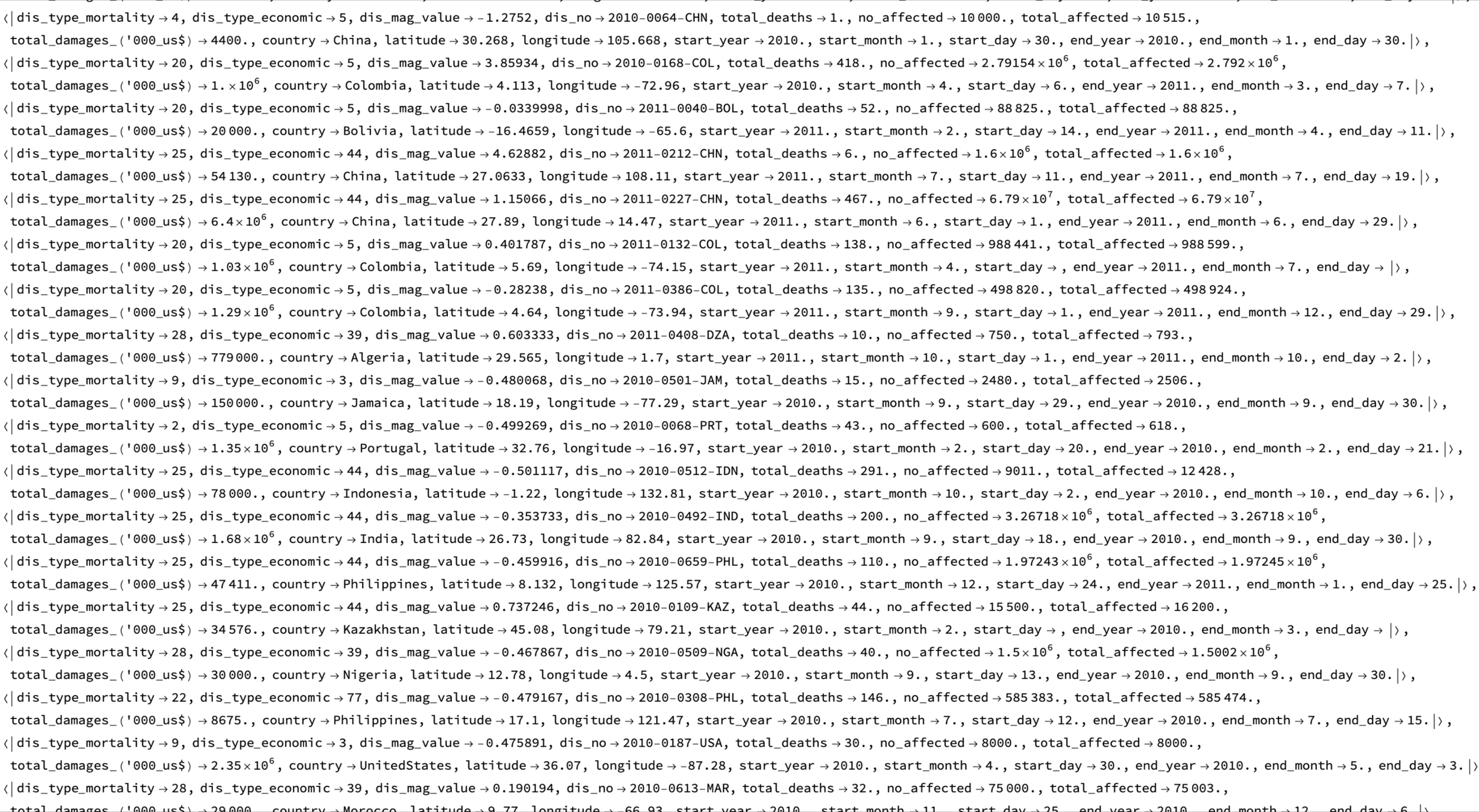
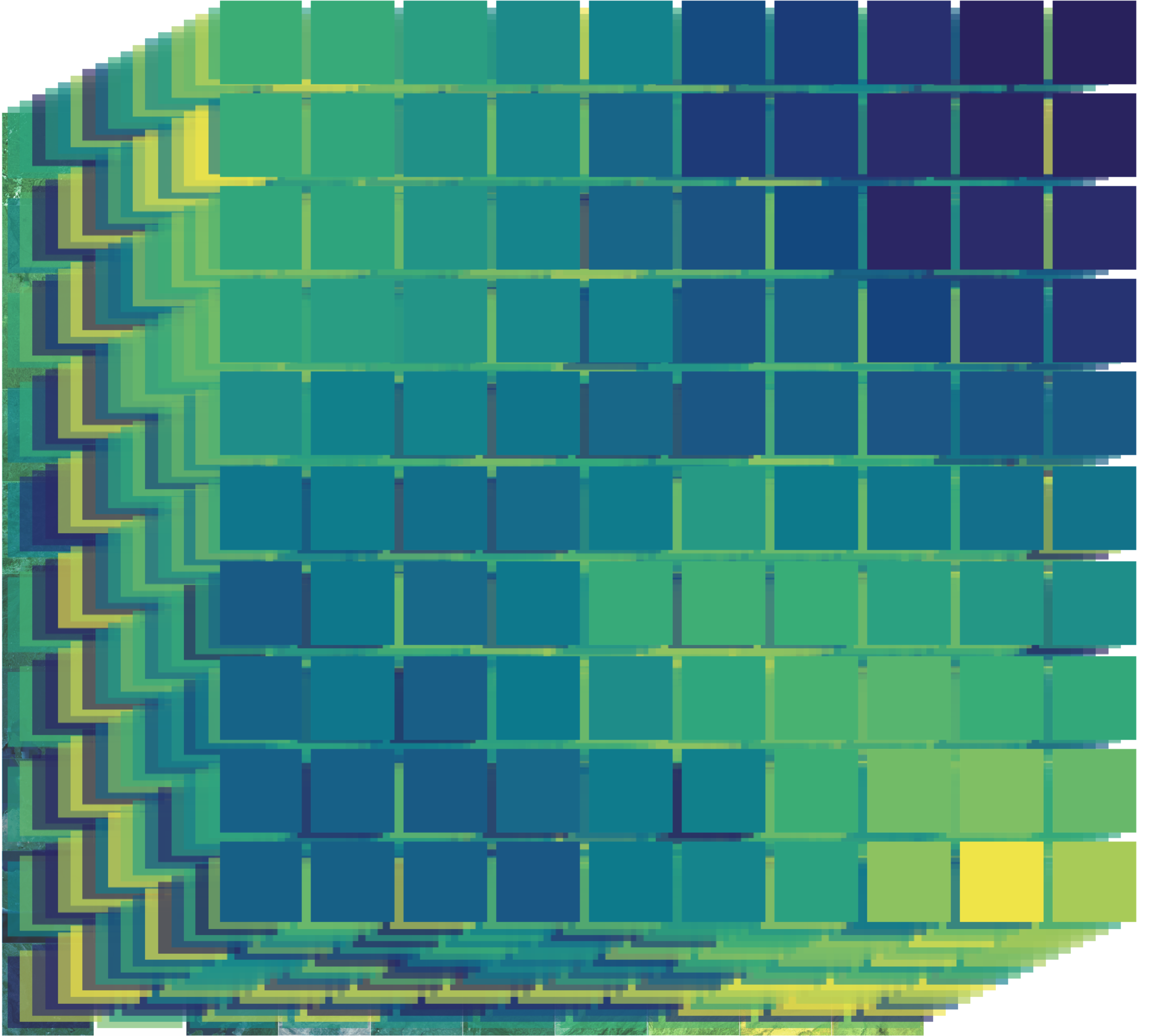

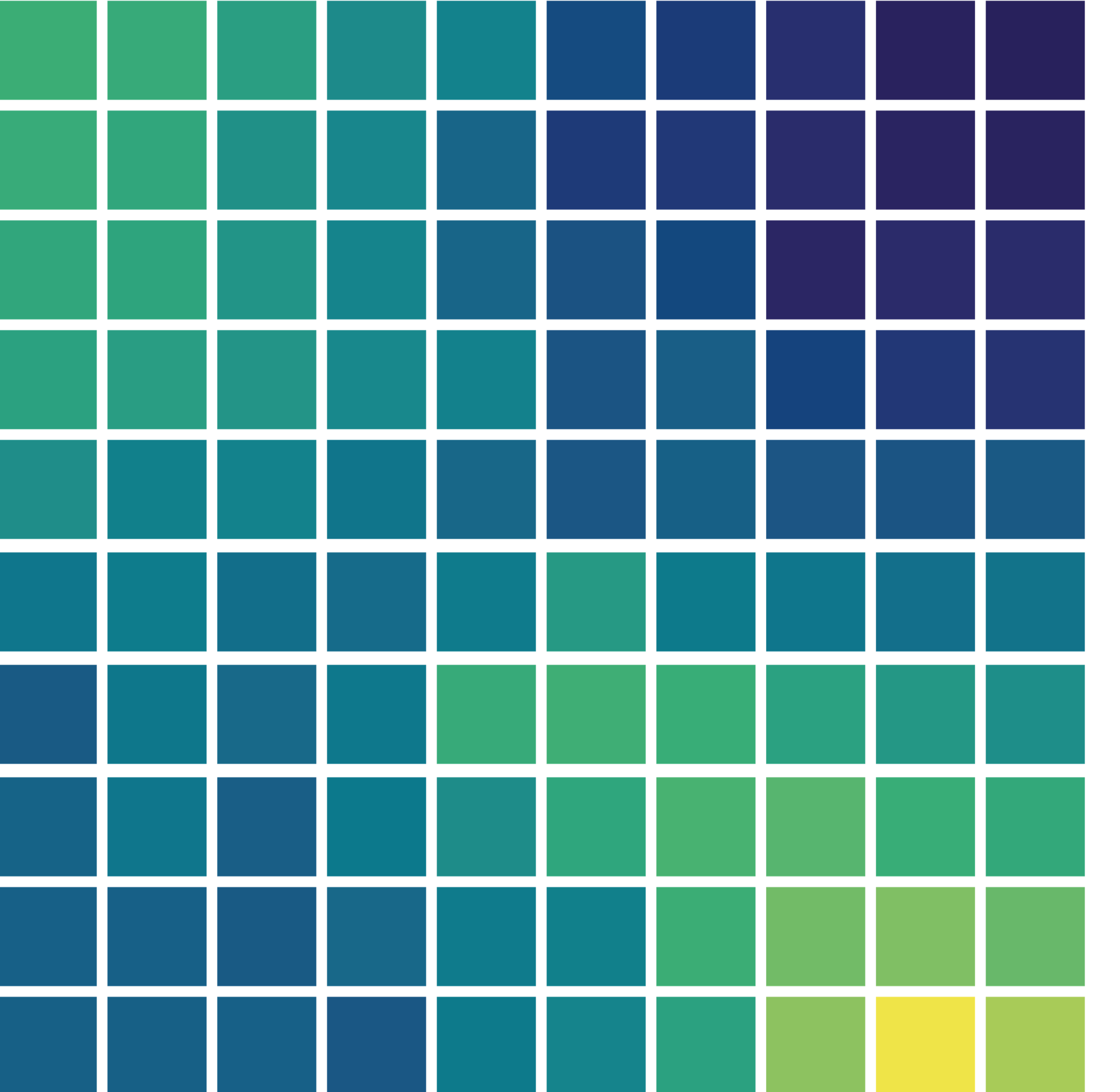
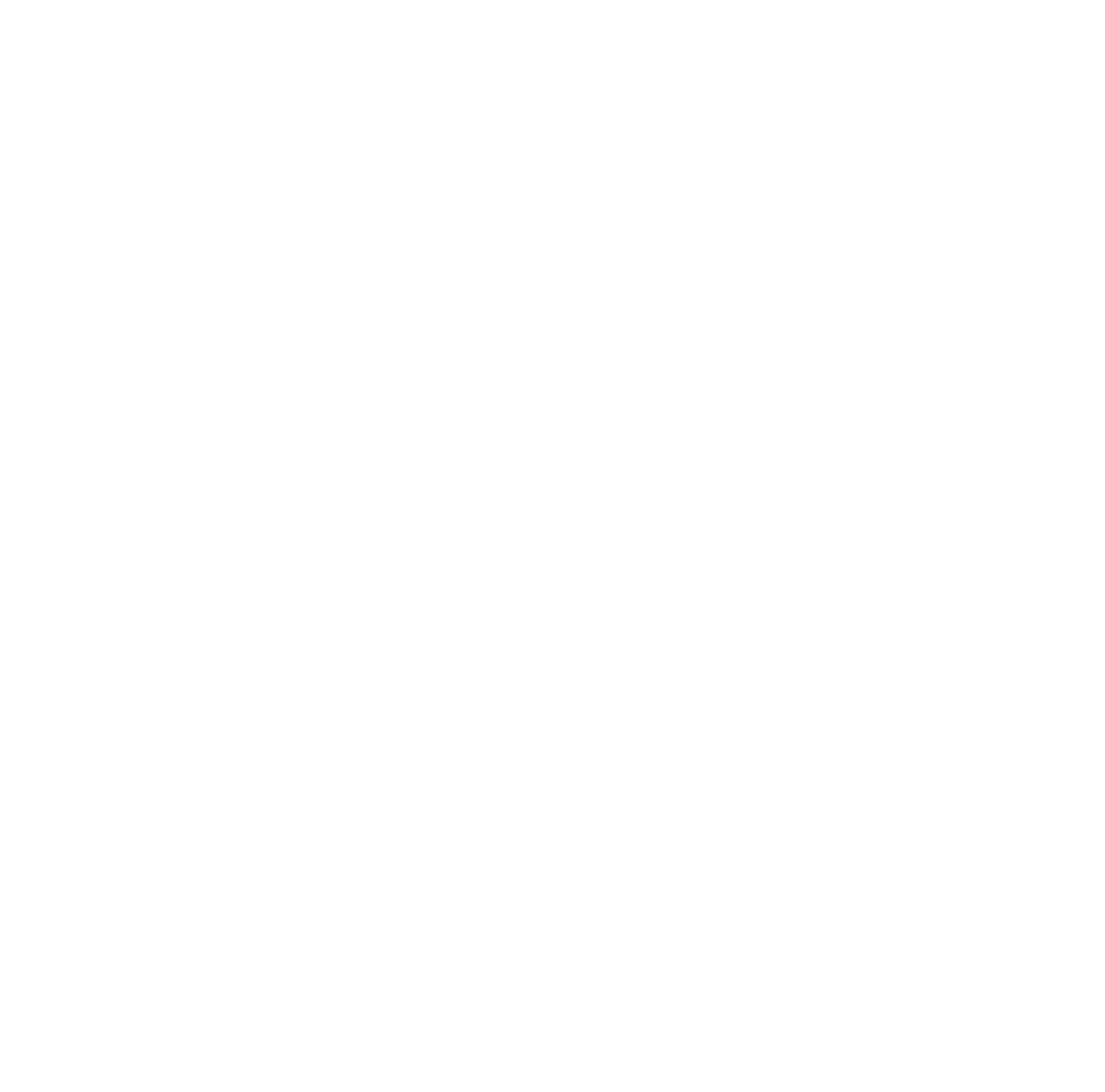
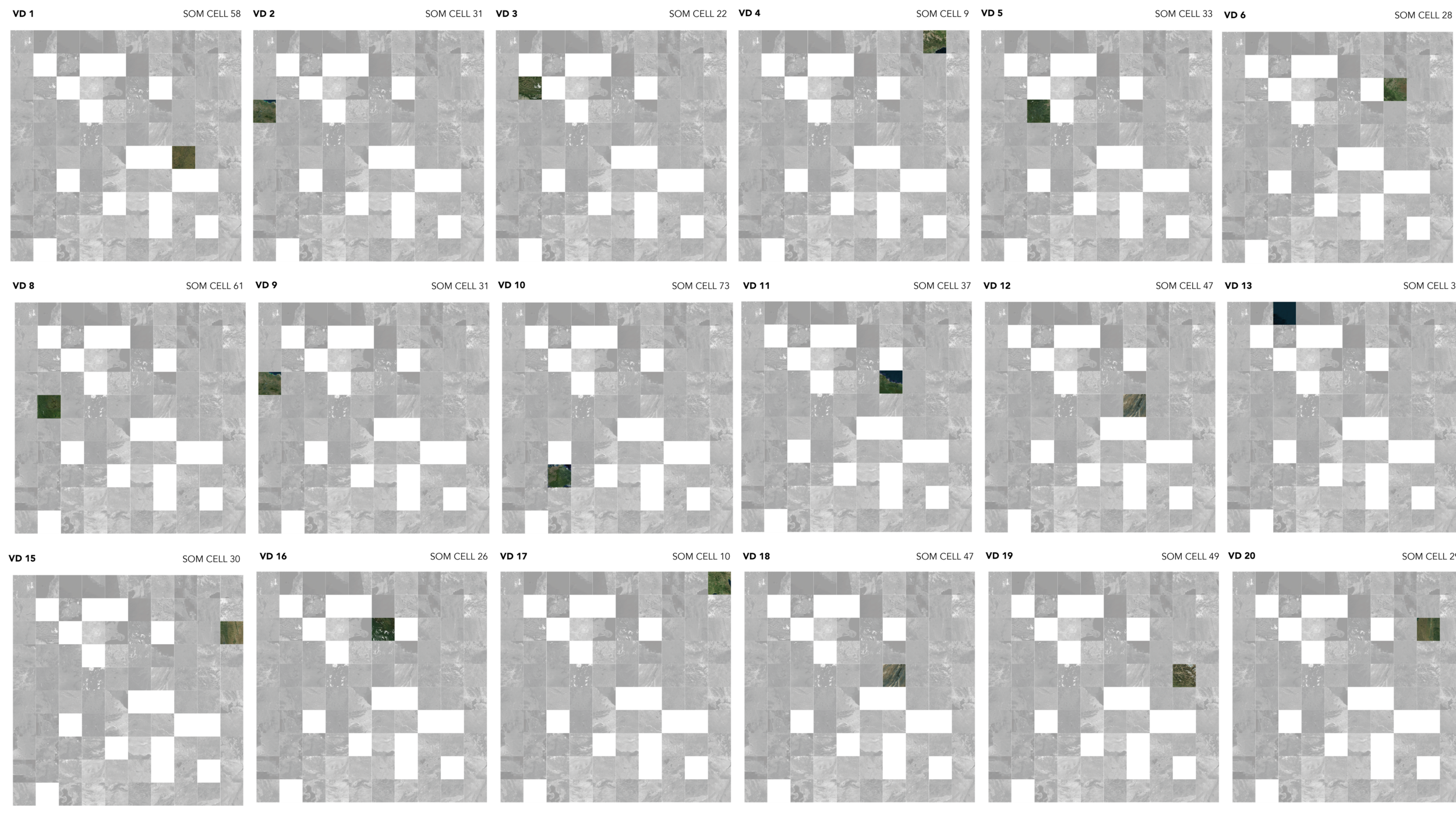
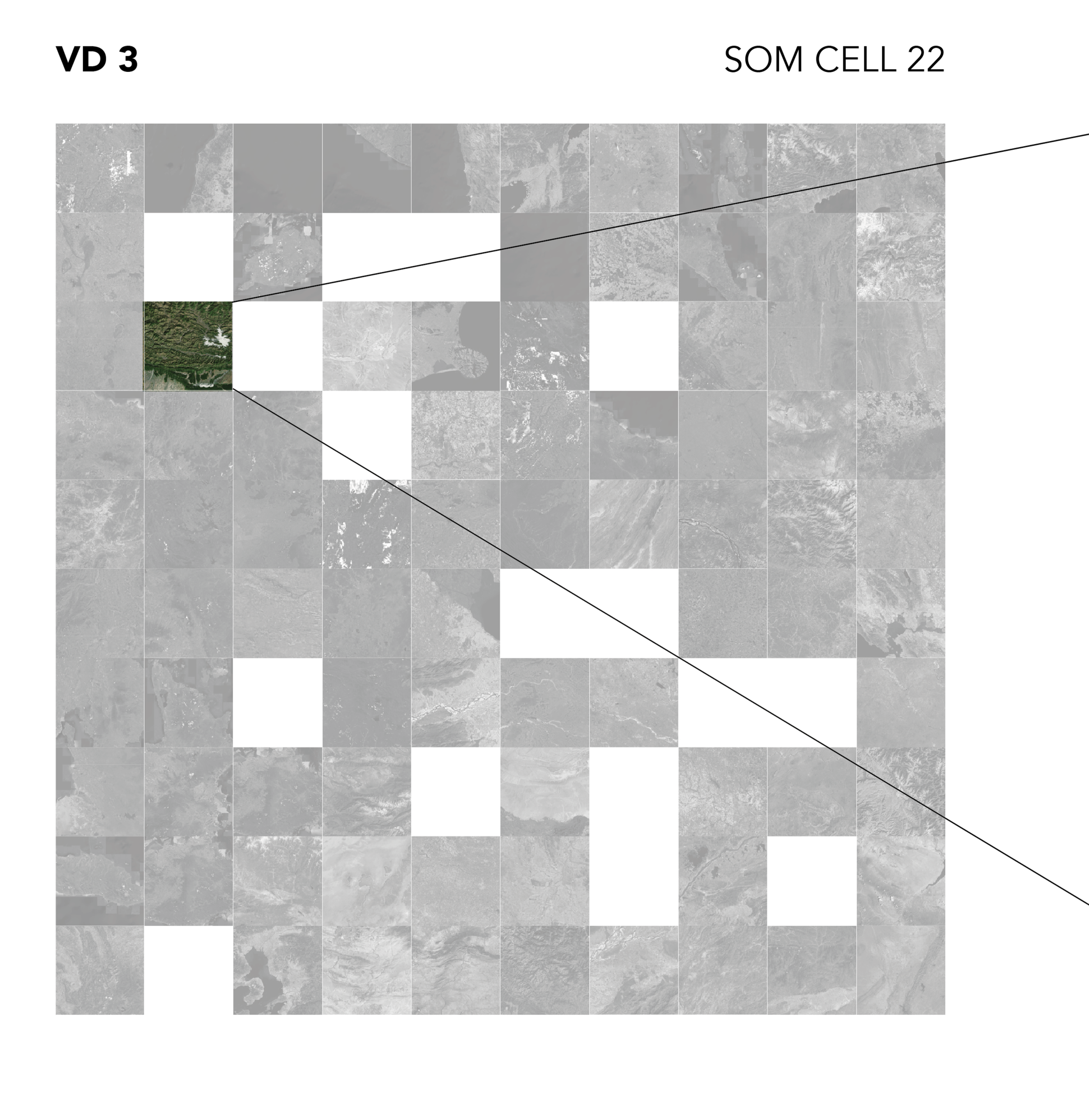
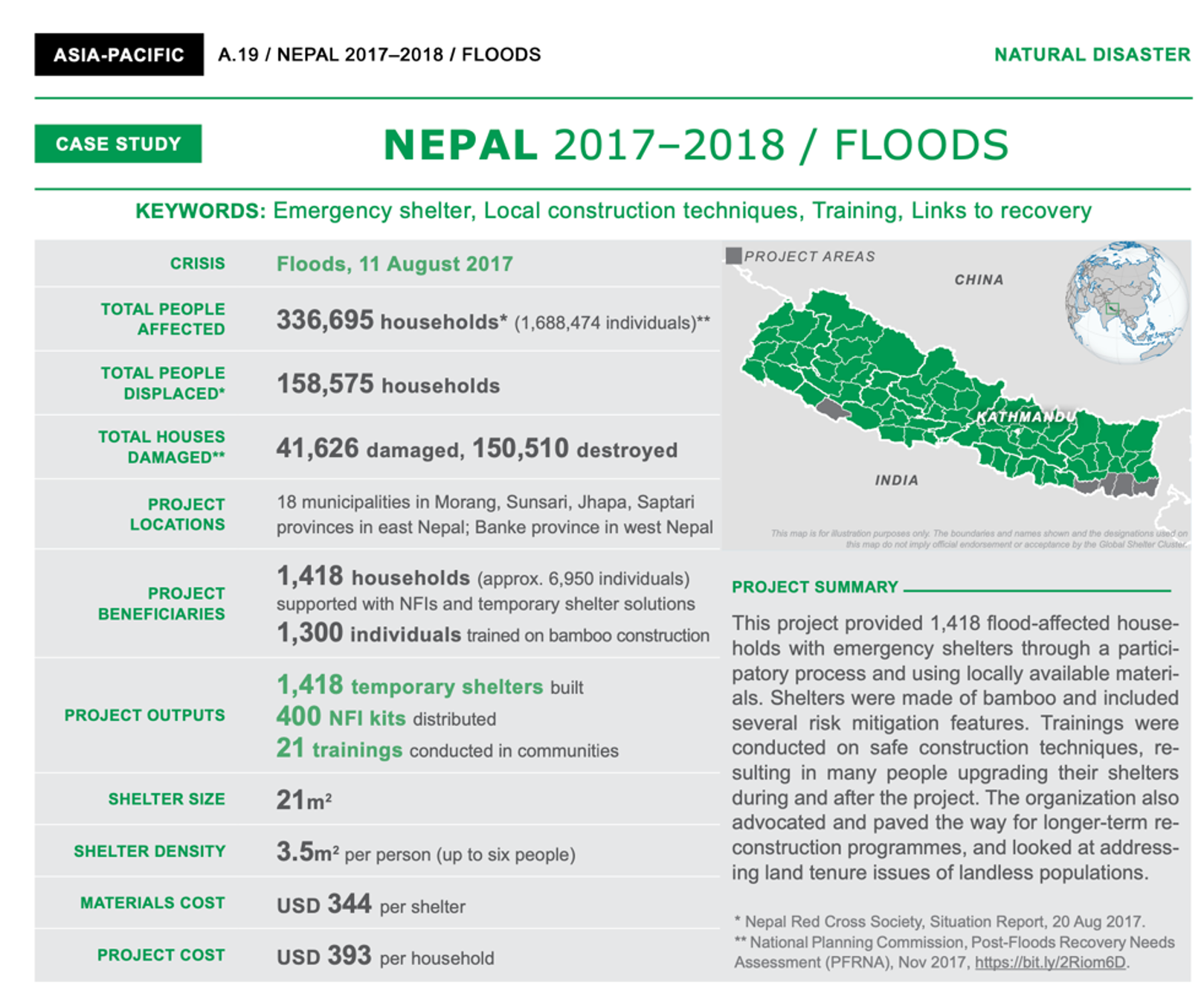

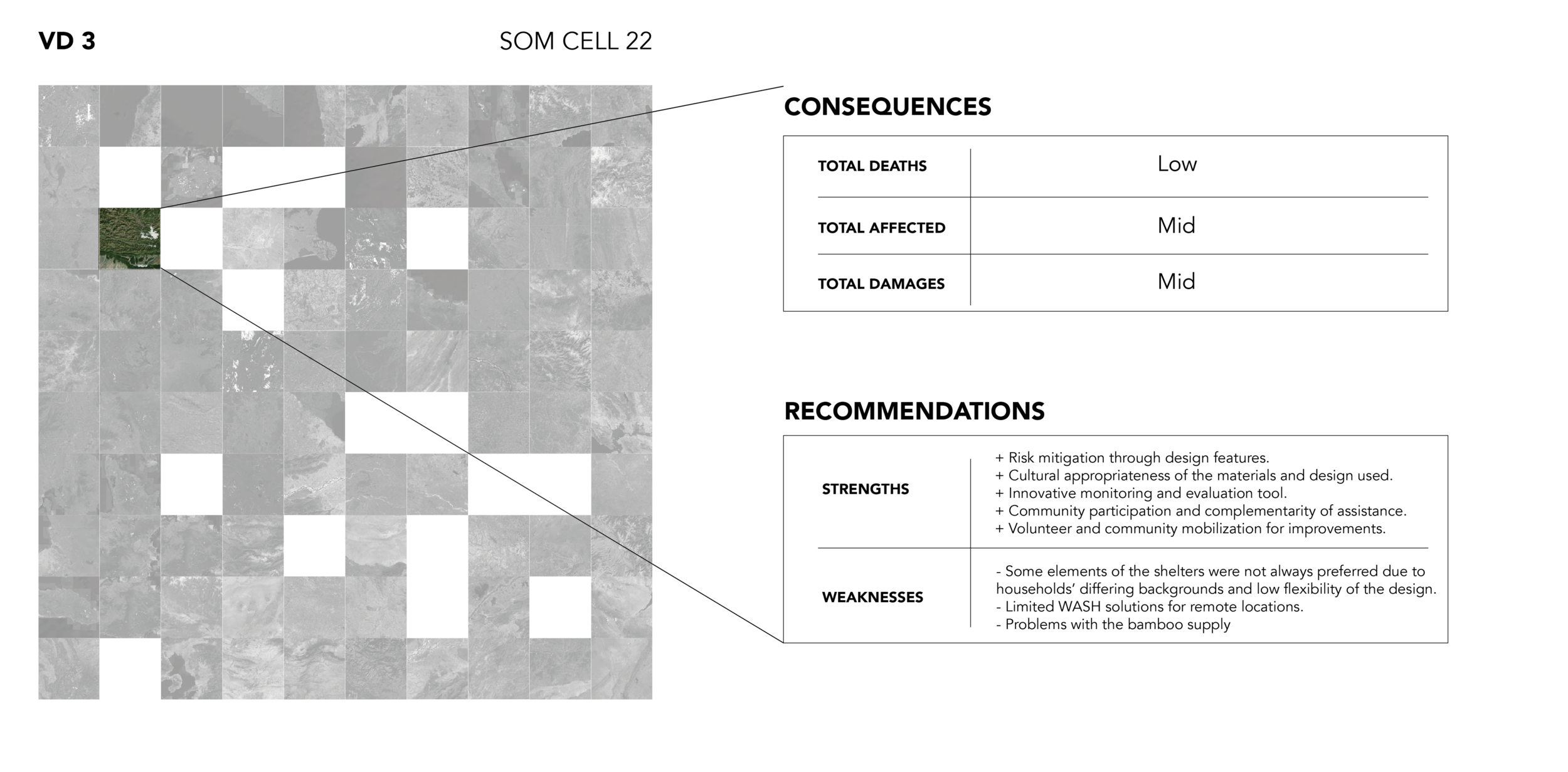
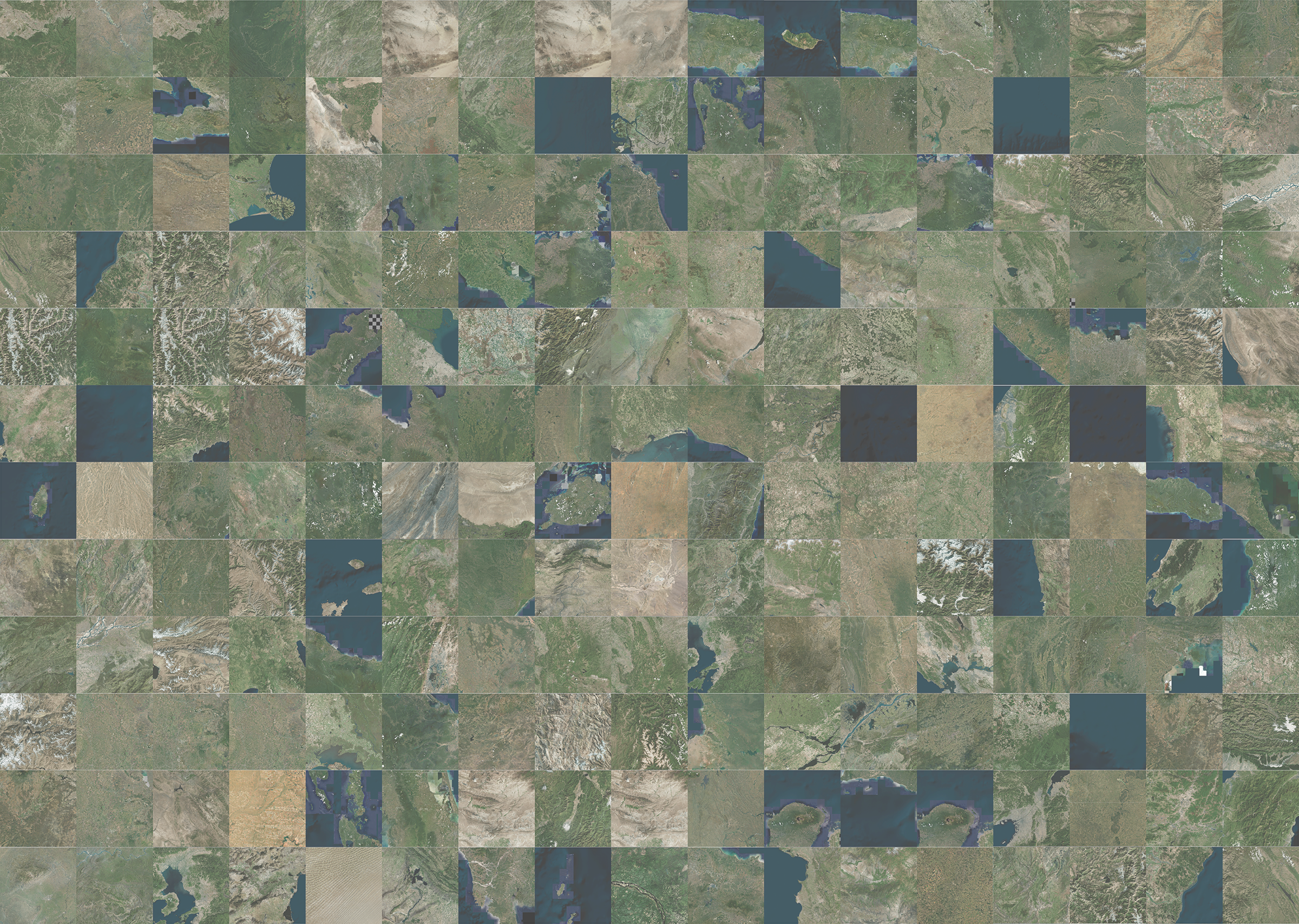