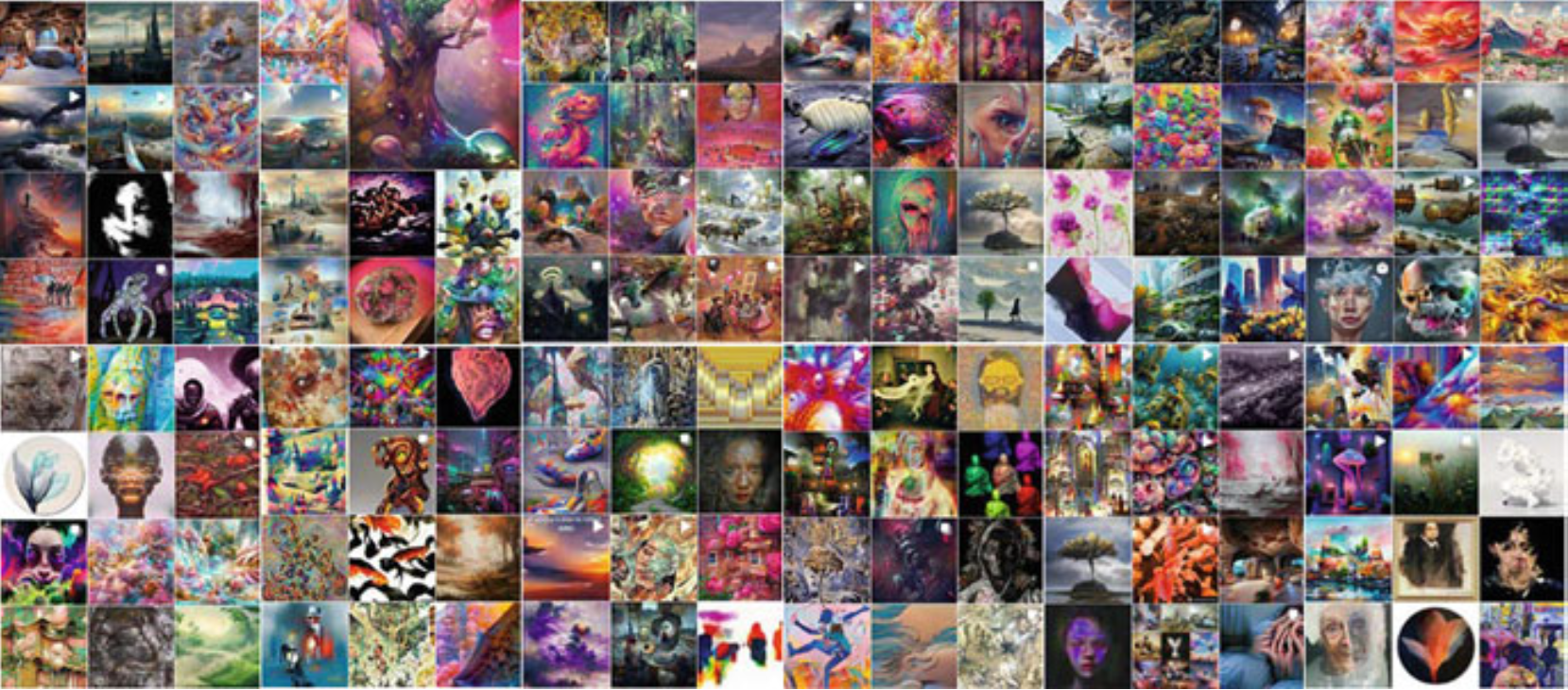
CAN ARTIFICIAL INTELLIGENCE MARK THE NEXT ARCHITECTURAL REVOLUTION?
Design Exploration in the Realm of Generative Algorithms and Search Engines
,
1 INTRODUCTION
Computing has evolved from a single central computer serving many people in the 1940s, to individual computers in the 1970s, and now to a world where each person uses multiple devices that continuously collect diverse data—known as Big Data. AI algorithms transform this digital data into numerical forms to detect patterns and make predictions. The origins of AI trace back to Ada Lovelace, who laid the groundwork for programming with her algorithm for the Analytical Engine, and to Alan Turing, whose Turing machine concept and Turing Test set the stage for modern computation and AI evaluation. This historical journey highlights the development of AI tools, including generative algorithms, which are now increasingly applied in fields like architecture to address both well-defined and creative, ill-defined tasks.
Fig. 1 Compilation of architectural drawings from the web
2. GENERATIVE ALGORITHMS AND ARTIFICIAL INTELLIGENCE
To comprehend generative algorithms, let us juxtapose them with discriminative learning. Like a classifier, a discriminative algorithm models itself based on observed data, making fewer assumptions about distributions but relying heavily on data quality (refer to Fig. 2, left). Logistic Regression serves as an example of such a discriminative algorithm. In contrast, generative algorithms aim to construct positive and negative models, envisioning a model as a “blueprint” for a class (refer to Fig. 2, right). These models delineate decision boundaries where one becomes more probable, effectively creating models for each class that can be employed in generations.
Fig. 2 An example of discriminative learning and generative learning
In supervised learning, both discriminative and generative algorithms rely on example data, using features and corresponding labels to predict outcomes for new inputs. This type of learning is one of four machine learning approaches, alongside unsupervised, semi-supervised, and reinforcement learning. The text also clarifies that while AI encompasses both ML and deep learning (DL), not all generative algorithms are ML, and not all ML algorithms are DL. A prime example is the Generative Adversarial Network (GAN), where a generator creates realistic data and a discriminator learns to distinguish generated data from real data, thereby refining the generator's output through their interactive training process.
Table 1 List of different AI generative algorithms using logic-and rule-based approach
Cellular Automata (CA): CA is a collection of cells on a grid of a specified shape that evolve over time according to a set of rules driven by the state of the neighbouring cells
Genetic algorithms (GA): GAs and genetic programming are evolutionary techniques inspired by natural evolutionary processes
Shape grammars (SG): SG is a set of shape rules that can be applied to generate a set or design language. The rules themselves are the descriptions of the generated designs.
L-systems (LS): LSs are mathematical algorithms known for generating factual-like forms with self-similarity that exhibit the characteristics of biological growth.
Agent-based models (ABM): ABM are often used to implement social or collective behaviors. Agents are software systems capable of acting autonomously according to their own beliefs.
Parametric design (PD): PD is a process based on algorithmic thinking that enables the expression of parameters and rules that, together, define, encode, and clarify the relationship between design intent and design response
The iterative process between the generator and discriminator in models like GANs continues to improve both components. Another example of generative AI is the diffusion model, used by systems like Midjourney and DALL-E, which creates complex content by diffusing information across neural network layers. These models excel at generating high-quality images from text prompts, capturing intricate patterns and details. The rise of these advanced generative models has sparked concerns among creative professionals, who worry that the powerful capabilities of AI, such as effortlessly translating text into novel visual outputs, might threaten the traditional roles of designers and architects.
Fig. 3 An example of the diffusion process. Diffusion occurs in multiple steps. The model learns how to remove noise from an image and the steps it took to clean it up. The trained noise predictor can handle a noisy image and denoise it by following the denoising steps learned in training, generating a new noise-free image out of pure noise paired with a text description
Fig. 4 Compilation of images from social networks with the AI art tag
Rather than viewing generative AI as a threat, it should be seen as a transformative force that encourages creatives to redefine their roles. While AI offers rapid ideation and efficiency, it lacks the intuition, contextual understanding, and nuanced insights that human designers bring. By combining human ingenuity with AI's capabilities, designers can focus on higher-level creative decisions and critical thinking. Additionally, the integration of AI in creative fields raises important ethical and societal questions, underscoring the need for a balanced, collaborative approach that preserves the unique contributions of human creators.
3 SEARCH ENGINES AND ARTIFICIAL INTELLIGENCE
The rapid advancement of AI algorithms and open-source datasets has significantly impacted industries like architecture. Open-source data enhances architectural projects, while big data is crucial in training generative algorithms (GA). While GA generates new information through interpolation, search engines (SE) provide relevant results by indexing keywords, making them vital for information retrieval and research in architecture. A focus on GA using diffusion models and Large Language Models (LLM) like ChatGPT highlights the importance of addressing AI 'hallucination'—unjustified responses. SE addresses this by relying on actual indexed data, offering a solution to hallucination issues. Effective dataset curation and personalization are key to overcoming challenges in project-specific inquiries.
4. METHODOLOGY
To describe how AI is revolutionizing architectural practice, this chapter presents examples of how the use of AI in various stages of design can enhance the creative capacity of a designer. First, it offers two examples of today’s most commonly used algorithms (DALL-e and Chat GPT). Second, it presents four examples of other AI algorithms leveraging search engines applied to architectural design.
4.1 IMAGES
Recent months have seen the ease with which architects embrace the mass production of images (Fig. 5). The former invites us to think that architects have taken on the passive role of curators of images. However, are they still the authors of those images? That is not the case, entirely. These images are not their creations but iterations of possible projects that serve to inspire future project ideas. Authorship of these images is shared with the researchers who create the algorithm, the people who produce the training data, and the designers who generate the images. That is, we must take responsibility for not assigning all design responsibilities to one algorithm and for ensuring that both the data used for training and the architecture of the algorithm are adequate to help us answer the question that every architectural project has because architecture is more complex than just the formal part that an image can capture; the final work results from all the agents involved in the production.
Fig. 5 Screenshot of Dall-e, a generative algorithm created by open AI
Fig. 6 Comparison matrix between Image and Prompt level of detail—artwork by Joel Esposito
To effectively use a diffusion model, one must understand how its two main inputs—the prompt and the control image—influence the output. The study explored various combinations of level of detail (LOD) for these inputs, with prompt LOD on the x-axis and image LOD on the y-axis. Higher prompt specificity enhances contextual shading, while more detailed control images yield finer features like fenestration. Due to the model's rapid output, the number of generation cycles was limited in the experiment, as additional processing time was negligible for practical architectural applications.
Fig. 7 Line with exploration from Light to Heavy, using the same text prompt—artwork by Joel Esposito
Continuing our investigation, let us focus on the image input and experiment with various line weights, ranging from light to heavy, with the same text prompt as input (Fig. 7). We notice a discernible correlation between stroke weight and the LOD. Notably, as the line weight increases, the LOD also increases. The algorithm perceives the thicker stroke as indicative of volume, subsequently introducing shadows and imbuing the image with a sense of perspective. When dealing with the control image as input, it becomes crucial to consider the intricacy of the detail invested in the drawing and the choice of line weight employed to depict various elements.
4.2 TEXT
Let us talk about another medium: text. A text allows designers to describe the design space and present ideas that they (the designers) still need to explore. In this case, we used ChatGPT to experiment. We prompted it with the following text, “write an architectural project similar to a Le-Corbusier project.” After a few seconds, the following text appeared:
This project is an architectural marvel that transcends time and trends, remaining true to the principles of modernism espoused by Le-Corbusier. The building presents an iconic silhouette characterized by sleek lines, geometric forms, and innovative use of raw materials such as concrete, steel, and glass. The open floor plan and large glazed walls offer an unparalleled connection to the outdoors, blurring the line between indoor and outdoor spaces and providing abundant natural light. Sustainability and environmental responsibility are key elements of this project, which specializes in using greenery and vegetation to create a harmonious balance between the built and natural environment. The minimalist color palette and industrial feel give the building a raw, modern feel, while the strategic placement of vegetation brings a touch of nature to the urban landscape. In short, this project is a contemporary interpretation of Le-Corbusier’s timeless design principles, offering an unparalleled living experience in a harmonious balance of form and function. Prepare to experience the ultimate fusion of style, sustainability, and innovation.
The text questions where creativity lies in using generative algorithms in architecture. It argues that writing an architectural brief should involve synthesizing design thinking—incorporating research on the place, user comfort, and needs—rather than simply listing a program. By analyzing generated text, architects can identify when the output deviates from established design philosophies, as seen in the example where the text does not align with Le Corbusier’s focus. Ultimately, architects must carefully formulate questions to control or leverage algorithmic "hallucinations" in order to preserve creative integrity in the design process.
5 OTHER EXAMPLES OF AI IN ARCHITECTURAL DESIGN
AI can assist architects and designers by enabling better decision-making, generating innovative ideas, and exploring previously unattainable design possibilities. Architecture involves a synthesis of form, functionality, needs, and comfort—not just generating images or descriptions. While the field has seen little change over the past 100 years, AI and data-driven methodologies introduce a new paradigm. These technologies allow architects to access millions of design solutions tailored to their preferences or aligned with a particular design style. Rather than replacing human creativity, AI supports it. Four projects will demonstrate how AI empowers architectural creativity without replacing the designer's expertise.
5.1 PROJECT 1
An AI search engine was used to organize over 12,000 images from social media to analyze the needs of a geographic location. Unsupervised AI algorithms, specifically the self-organizing maps algorithm, clustered similar images and created a grid representing the site’s needs, capturing users' sentiments about the place. This approach enables designers to explore the site in a collective way, beyond traditional analysis methods such as site visits and interviews, offering a fresh perspective on site analysis that incorporates user activities and perceptions.
Fig. 8 Top left, representation of the collected social network data. On the top right are the commonalities achieved with AI clustering algorithms; on the bottom are the atmospheric images—artwork by Sarah Gurevitch
5.2 PROJECT 2
Figure 9 illustrates how designers transformed atmospheric images and text into a collaborative design narrative. They began by using a self-organizing map to cluster social media data into a grid representing site-related concepts. These images and concepts were then fed into DALL‑E to automatically generate new images. Next, designers employed ALICE, an AI search engine based on architectural treatise libraries, to link these generated images with similarly themed concepts by curating relevant paragraphs from treatises. This process culminated in a collaborative exercise—reminiscent of a joint drawing and writing project—in which historical and contemporary voices merged to articulate a comprehensive architectural vision.
Fig. 9 On the left, the title of the work and concepts describe the design intentions; each concept was developed with ALICE to find quotes from prominent authors. On the right, atmospheric images are generated with diffusion models and social network data—artwork by Marc Kiener
5.3 PROJECT 3
This project introduces an innovative architectural design workflow that integrates Big Data, AI, and storytelling. It aggregates 20,000 social media posts (text and images) and 9,000 3D architectural models to provide insights into user needs and site characteristics. Using an unsupervised clustering algorithm (self-organizing maps), the data—including 3D objects—is organized into grids that allow architects to personalize their searches and enrich their design ideas. The resulting conceptualizations are then implemented in game engines and tested in VR/AR environments, promoting a design process informed by hyper-connected digital objects rather than starting from scratch.
5.4 PROJECT 4
Focusing on the fusion of architecture and structural engineering, this project redefines structural design by incorporating AI to interpret semantic inputs. It challenges the traditional deterministic form-finding approach by allowing designers to use textual inputs to generate spatial structures in static equilibrium. The outcome is Text2Form3D, an ML-based framework that combines Natural Language Processing (NLP) to convert text into numerical features with the Combinatorial Equilibrium Modeling (CEM) algorithm. This synergy enables the system to propose design parameters based on the designer’s semantic requests, fostering a more creative and open exploration of structural design.
Fig. 10 SOM examples of images, text, and 3D objects
Figure 13 illustrates the Text2Form3D initial pipeline. In the first step, the CEM algorithm generates a dataset comprising diverse structural forms with randomly initialized design parameters. CEM, an equilibrium-based form-finding algorithm rooted in vector-based 3D graphic statics, sequentially constructs the equilibrium form of a structure based on given topology and metric properties. The dataset encompasses both the design parameters and the resultant forms. In the subsequent step, self-organizing maps (SOM) cluster the generated forms. The SOM training uses user-defined quantitative criteria to capture formal metric characteristics. Moving to the third step, the designer employs the trained SOM to label numerous design options using vocabulary sourced from architectural and structural practice competition reports. These labels, manually assigned to each form, create data for training a machine learning algorithm.
Fig. 11 Set of 3D objects as conceptual architectural ideas—artwork by Natalie Bergeron and Stephanie Roberts
Fig. 12 Staged scenographic storytelling settings
The workflow converts text labels into numerical vectors using word embedding, capturing semantic similarities. A deep neural network is then trained to map these text vectors to CEM design parameters, enabling the generation of design inputs from new text. Experimentally, this approach was used to create towers, with four distinct clusters of 3D forms emerging from different adjectives. Each cluster exhibits unique geometric characteristics, confirming that the method effectively translates semantic information into varied yet consistent geometries, with normally distributed random vectors facilitating exploration of design variations.
Fig. 13 Summary of tasks 1 and 2 of Text2Form3D, an algorithm that is based on a deep neural network algorithm that couples word embeddings, a natural language processing (NLP) technique, with Combinatorial Equilibrium Modeling (CEM), a shape search method based on graph statics
Fig. 14 Four examples of generated structures with different text inputs
The workflow highlights how human-AI collaboration can generate 3D structural forms by combining descriptive text and quantitative parameters. By integrating a text-based AI engine with computational graphic statics, the study demonstrates how structural forms can emerge from both static equilibrium and the designer’s semantic interpretation. This approach transforms the generative algorithm into an adaptive tool that tailors suggestions to individual design preferences, shifting AI’s role from a simple input-output machine to a system that translates text into spatial geometries.
6. DISCUSSION AND CONCLUSION
AI is transforming design by generating endless solutions, yet its true value depends on addressing relevant design challenges through human collaboration. While AI can compute and predict faster than designers, it cannot replace the critical and creative thinking required for effective design. Researchers are advancing AI from a mere drawing tool to an intelligent design partner—currently, AI is used to generate realistic 2D images from text, but design inherently involves multidimensional aspects like user preferences, spatial perception, and site-specific requirements.
Architects should leverage AI to enrich their designs by integrating complex layers of information beyond traditional 2D representations, balancing novelty with familiarity. Ultimately, as with any instrument, the impact of AI in design depends on the human designer’s skill and ability to tune the technology to meet nuanced, multidimensional design goals.
Fig. 15 Thematic modeling of architectural design and artificial intelligence: 20,000 scientific articles on architectural design. The words at the bottom are the most frequently used terms in 2000 artificial and architectural design articles. The highlight in the cell represents how often that word was used in the article. The words ai, data, and machine are used less frequently. The former clearly shows that this topic is new and that no established research vector exists. This means that now is the time to explore how to proceed
AI will enhance rather than replace our roles by augmenting our design and production capabilities. It can adapt to individual preferences and serve as a suggestion engine, aiding both well-defined and creative, ill-defined tasks. Although AI can generate images, text, and 3D objects, it still lacks the human touch, critical thinking, empathy, and cultural sensitivity intrinsic to architecture. Ultimately, architects' deep expertise in design, history, and culture remains irreplaceable, with AI acting as a tool to inspire and accelerate the creative process without substituting human intuition.
Gallery

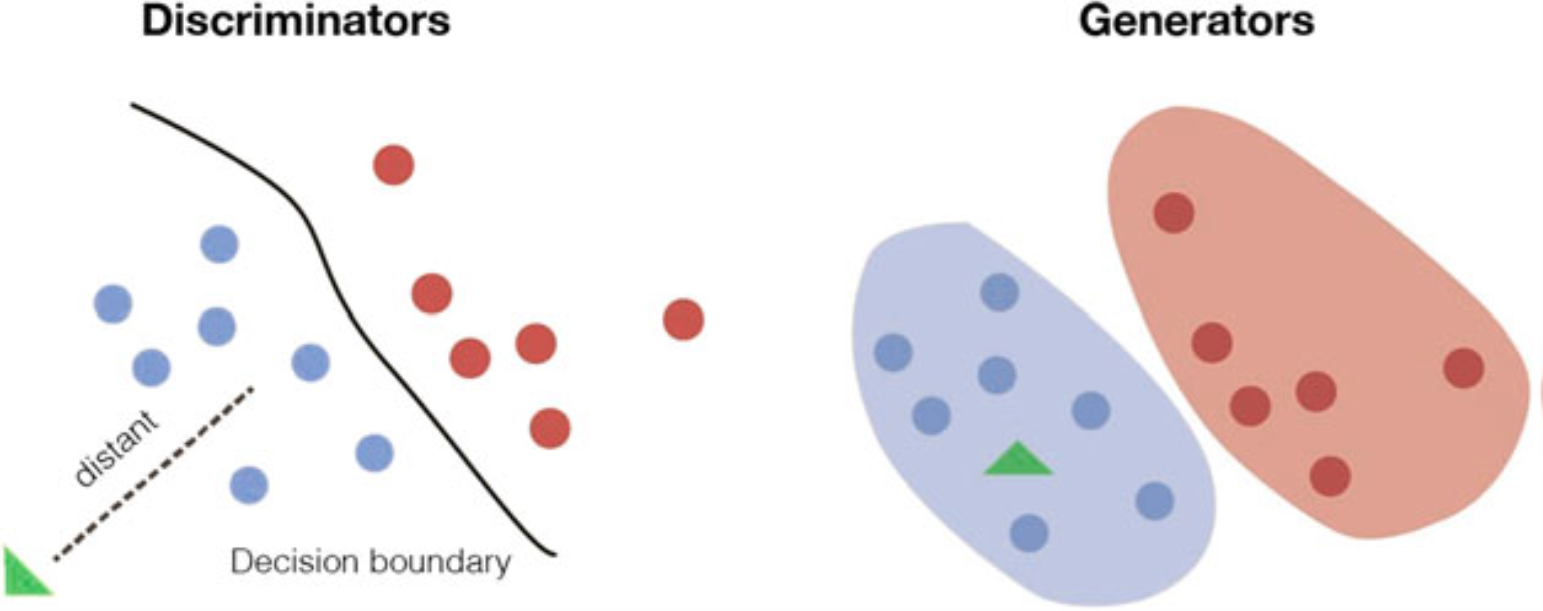
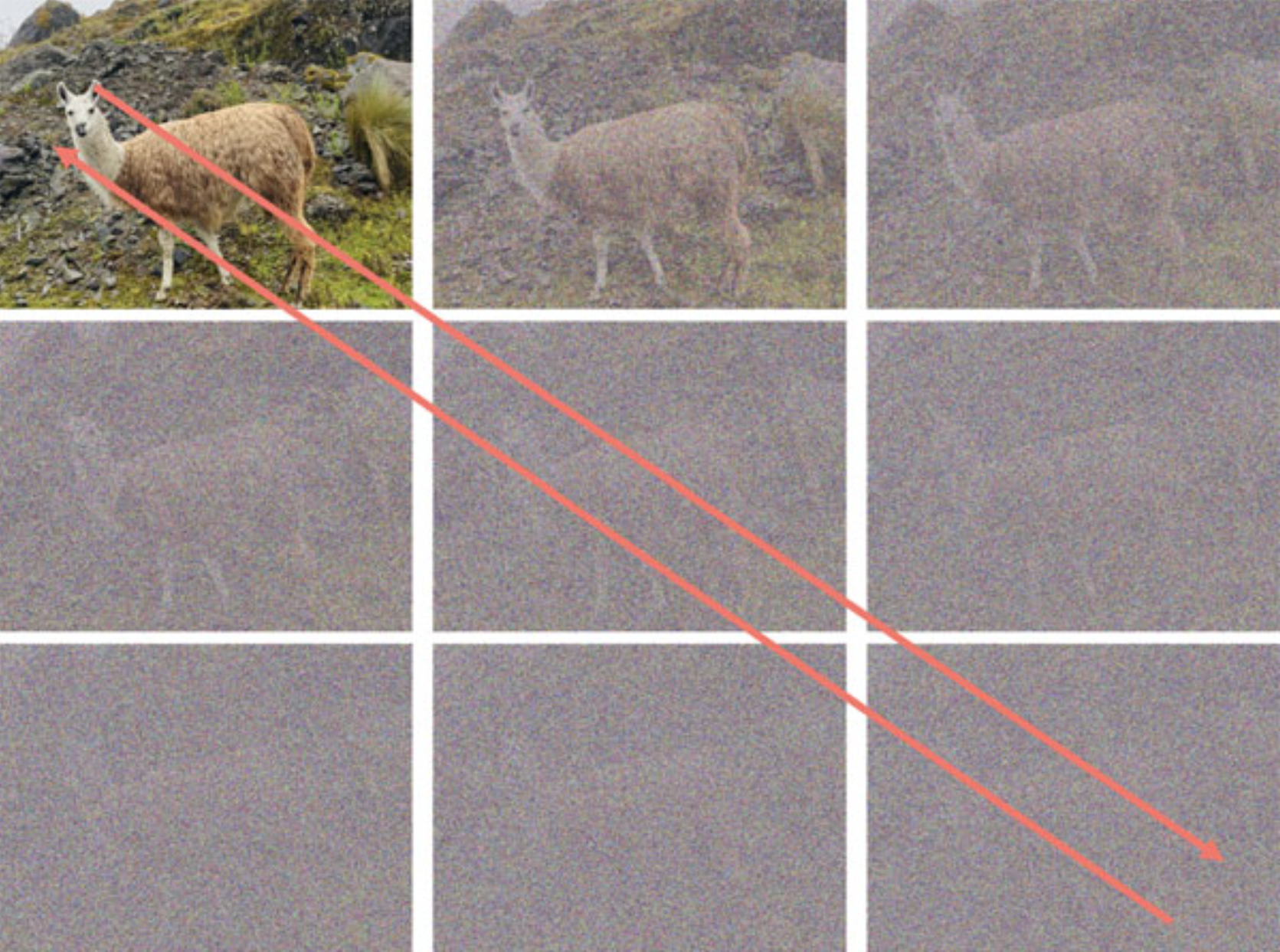
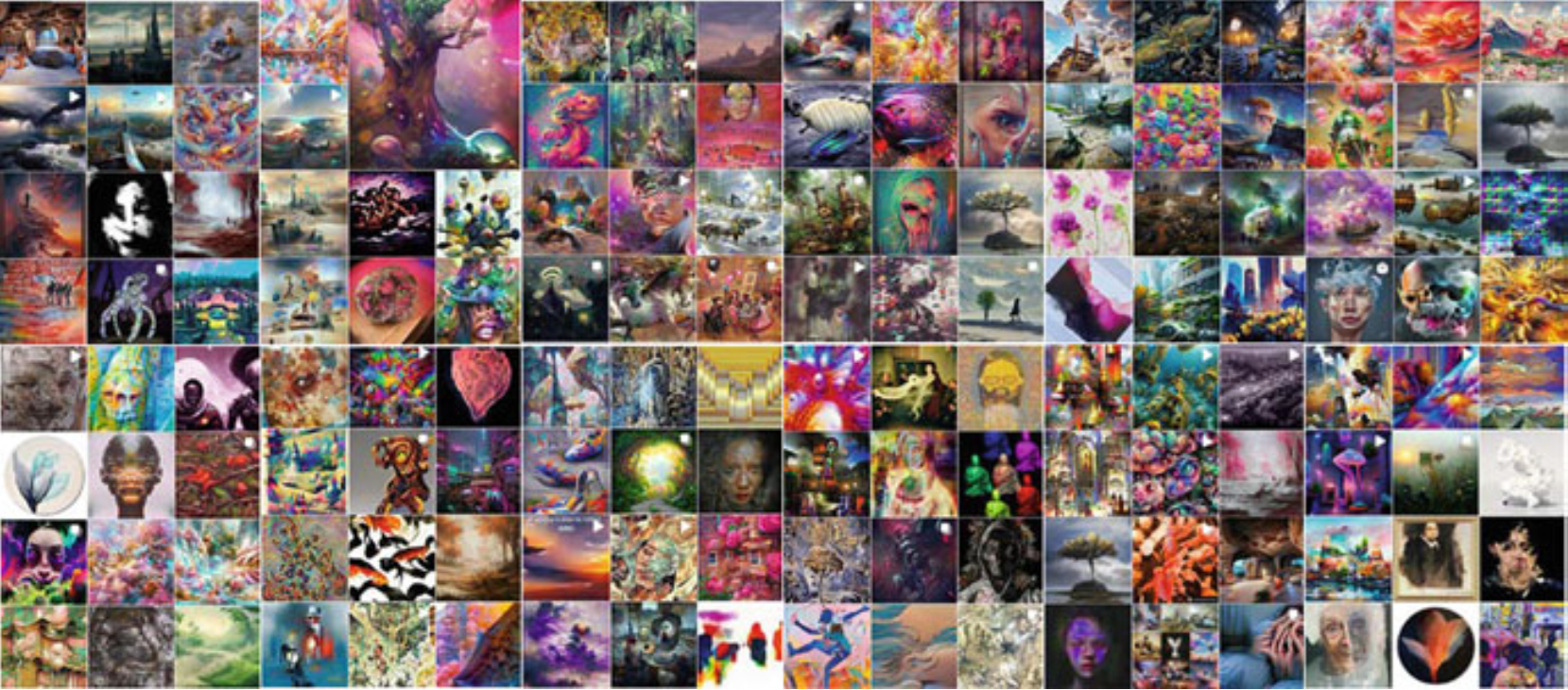


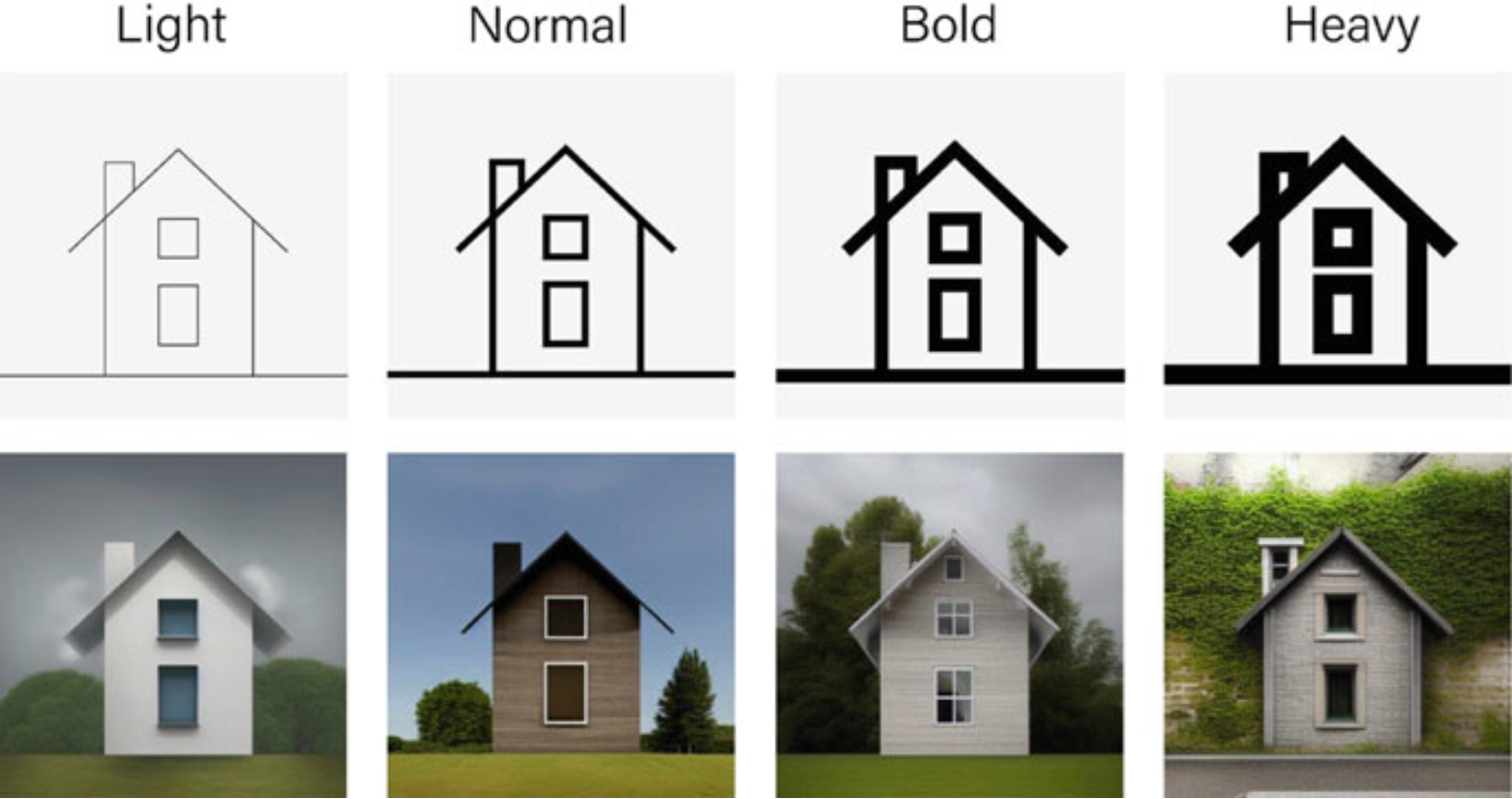
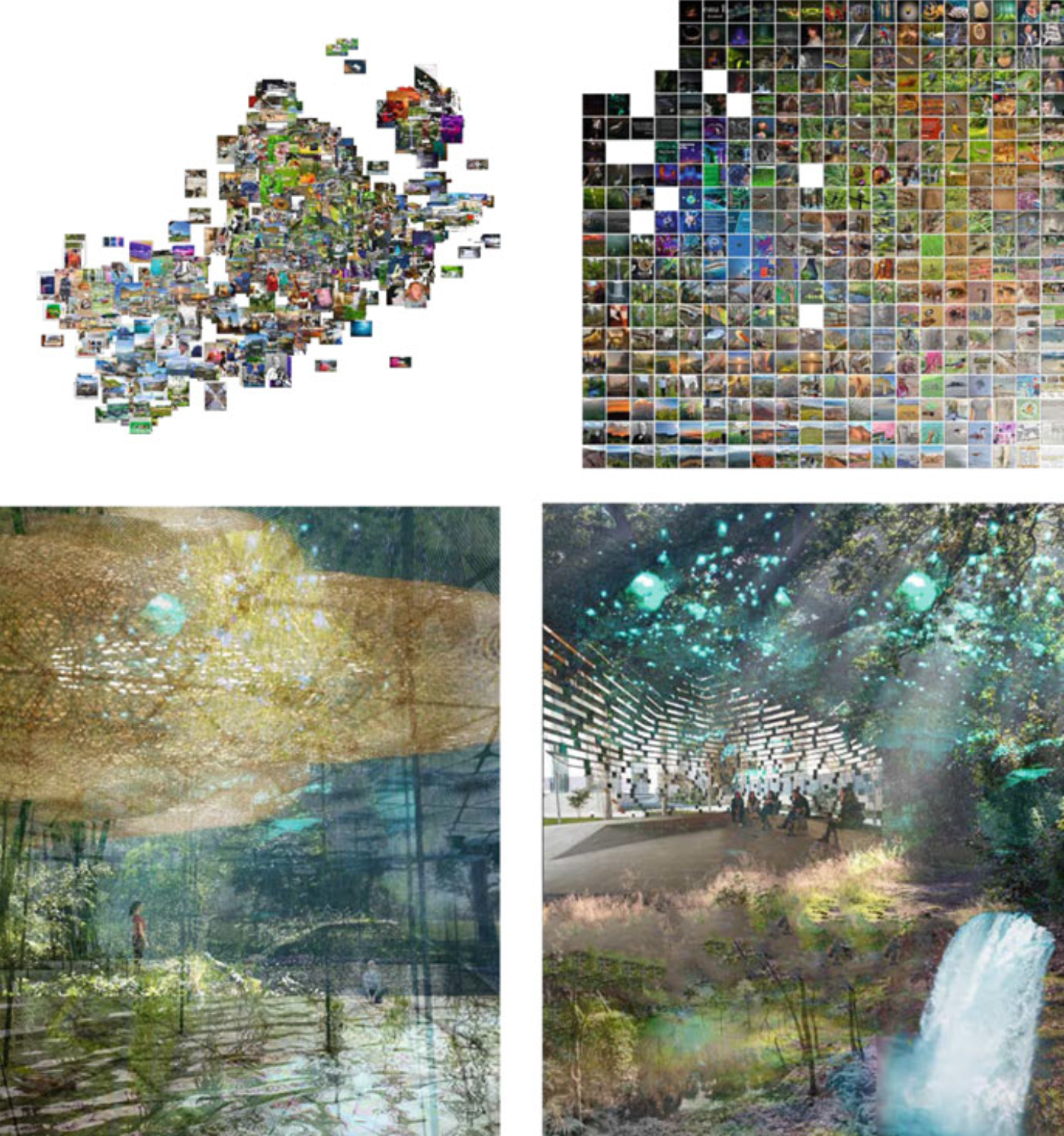
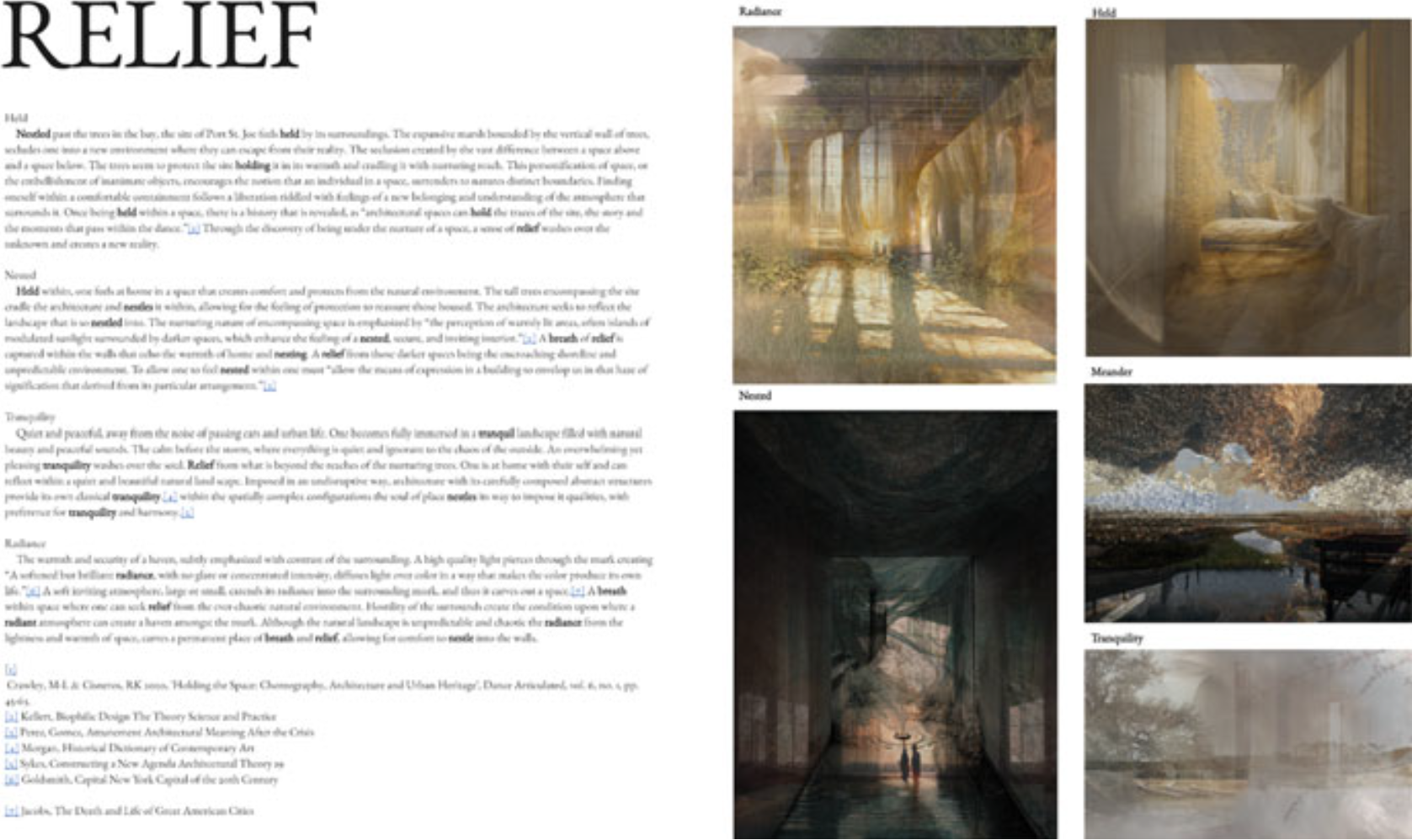
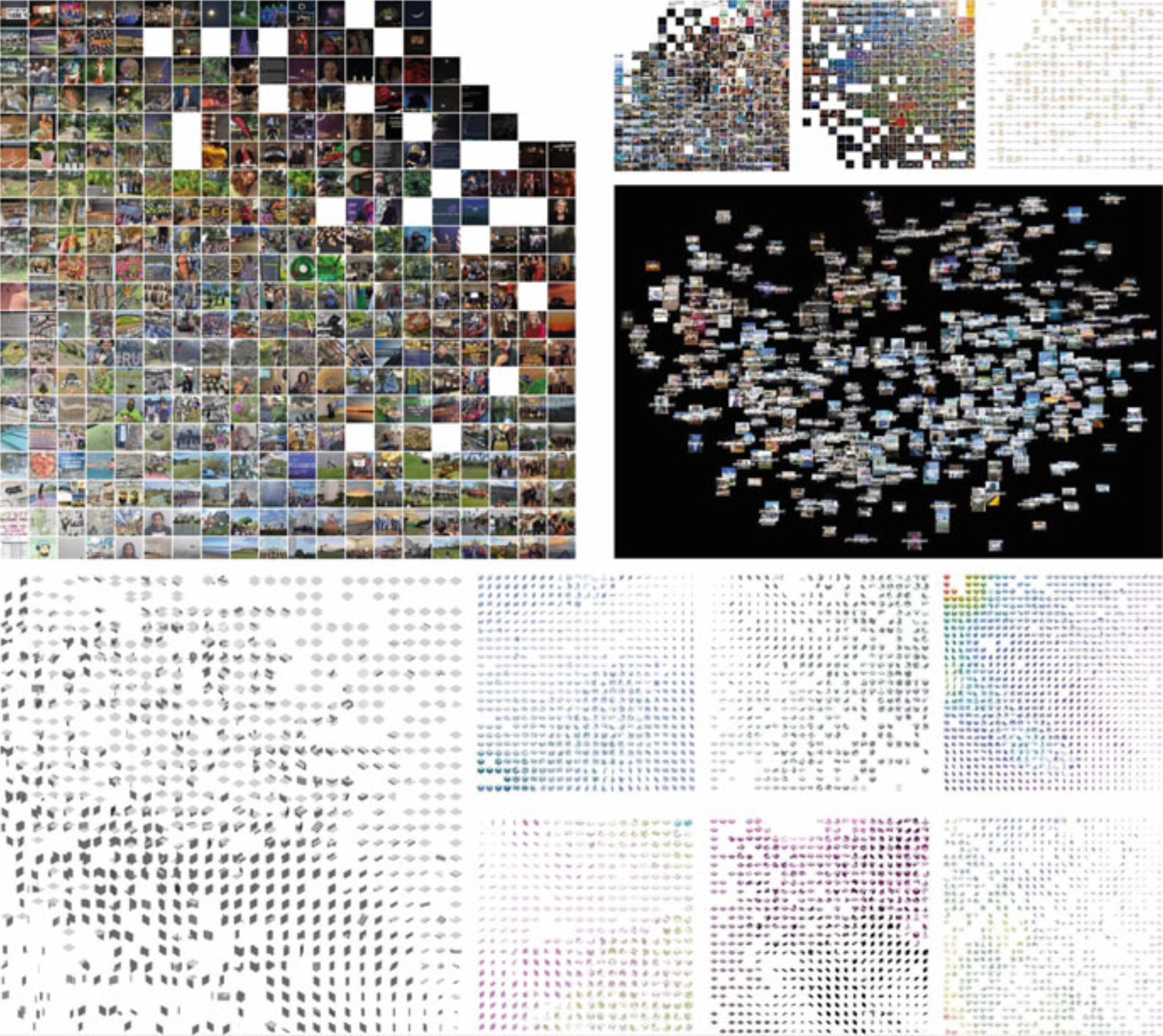


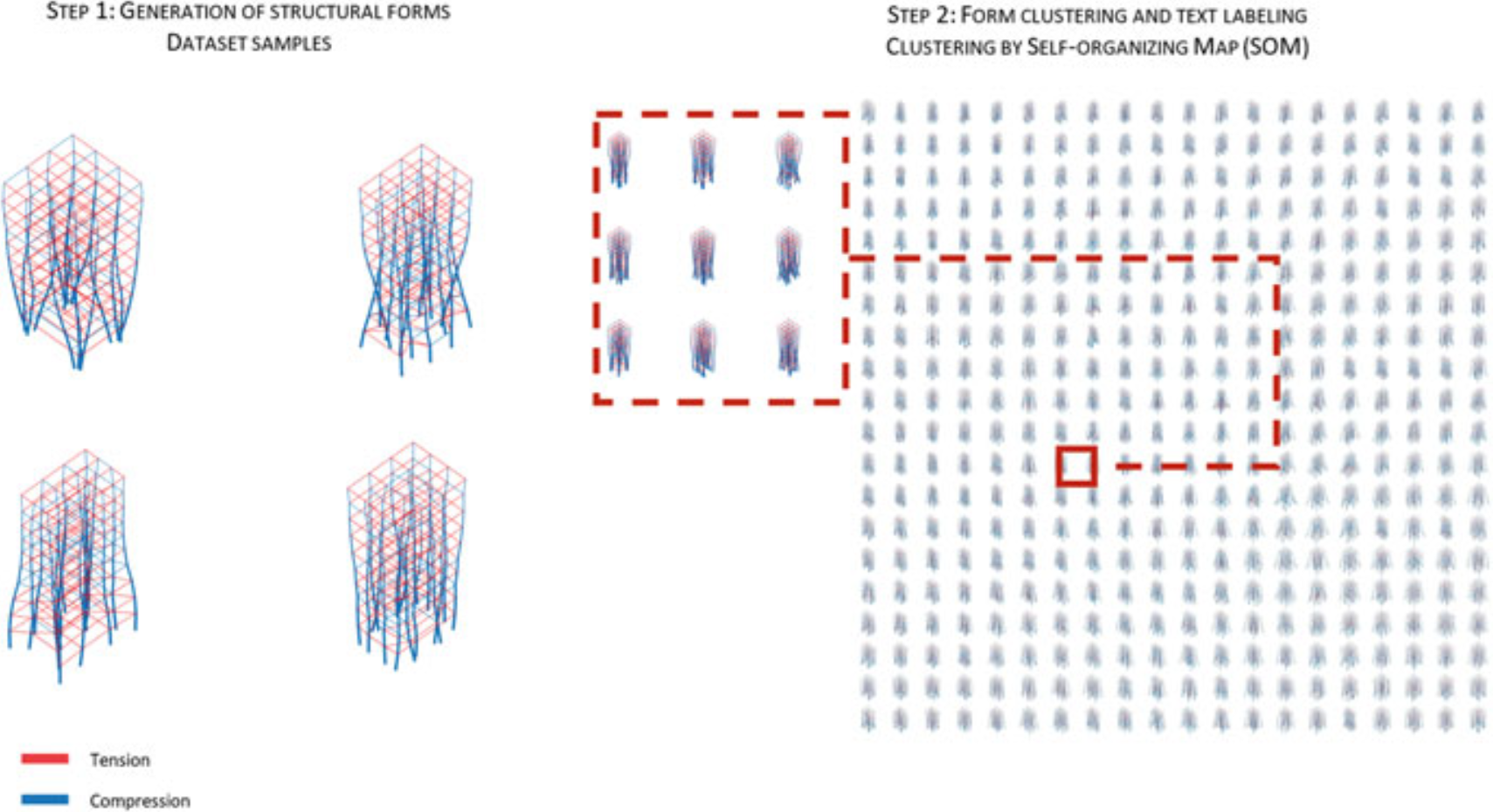

